The relationship between genomics and privacy-enhancing technologies (PETs) has been an intense one for the better part of the last decade. Ever since Wang et al.’s paper, “Learning your identity and disease from research papers: Information leaks in genome wide association study”, received the PET Award in 2011, more and more research papers have appeared in leading conferences and journals. In fact, a new research community has steadily grown over the past few years, also thanks to several events, such as Dagstuhl Seminars, the iDash competition series, or the annual GenoPri workshop. As of December 2017, the community website genomeprivacy.org lists more than 200 scientific publications, and dozens of research groups and companies working on this topic.
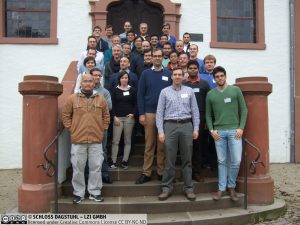
Progress vs Privacy
The rise of genome privacy research does not come as a surprise to many. On the one hand, genomics has made tremendous progress over the past few years. Sequencing costs have dropped from millions of dollars to less than a thousand, which means that it will soon be possible to easily digitize the full genetic makeup of an individual and run complex genetic tests via computer algorithms. Also, researchers have been able to link more and more genetic features to predisposition of diseases (e.g., Alzheimer’s or diabetes), or to cure patients with rare genetic disorders. Overall, this progress is bringing us closer to a new era of “Precision Medicine”, where diagnosis and treatment can be tailored to individuals based on their genome and thus become cheaper and more effective. Ambitious initiatives, including in UK and in US, are already taking place with the goal of sequencing the genomes of millions of individuals in order to create bio-repositories and make them available for research purposes. At the same time, a private sector for direct-to-consumer genetic testing services is booming, with companies like 23andMe and AncestryDNA already having millions of customers.
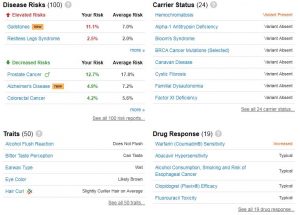
On the other hand, however, the very same progress also prompts serious ethical and privacy concerns. Genomic data contains highly sensitive information, such as predisposition to mental and physical diseases, as well as ethnic heritage. And it does not only contain information about the individual, but also about their relatives. Since many biological features are hereditary, access to genomic data of an individual essentially means access to that of close relatives as well. Moreover, genomic data is hard to anonymize: for instance, well-known results have demonstrated the feasibility of identifying people (down to their last name) who have participated in genetic research studies just by cross-referencing their genomic information with publicly available data.
Overall, there are a couple of privacy issues that are specific to genomic data, for instance its almost perpetual sensitivity. If someone gets ahold of your genome 30 years from now, that might be still as sensitive as today, e.g., for your children. Even if there may be no immediate risks from genomic data disclosure, things might change. New correlations between genetic features and phenotypical traits might be discovered, with potential effects on perceived suitability to certain jobs or on health insurance premiums. Or, in a nightmare scenario, racist and discriminatory ideologies might become more prominent and target certain groups of people based on their genetic ancestry.
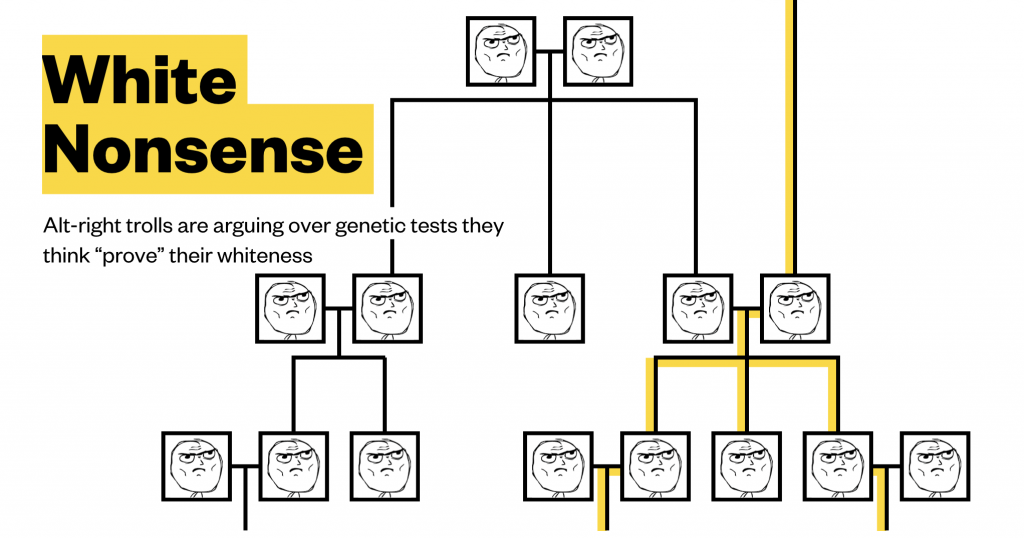
Making Sense of PETs for Genome Privacy
Motivated by the need to reconcile privacy protection with progress in genomics, the research community has begun to experiment with the use of PETs for securely testing and studying the human genome. In our recent paper, Systematizing Genomic Privacy Research – A Critical Analysis, we take a step back. We set to evaluate research results using PETs in the context of genomics, introducing and executing a methodology to systematize work in the field, ultimately aiming to elicit the challenges and the obstacles that might hinder their real-life deployment.
During the summer, we retrieved and examined about 200 papers listed on genomeprivacy.org. We decided to exclude papers on attacks and on privacy quantification as we wanted to focus on the use of PETs in genomics – i.e., on defenses. We were able to group the research results in several “categories,” ranging from privacy-respecting versions of ancestry or disease predisposition tests, to privacy in the context of sharing or outsourcing genomic data, as well as statistical research such as genome-wide association studies.
Then, we selected what we consider the 21 most representative papers in the context of testing, storing, and sharing genomic data. The list of the papers can be found here.
Are we going in the right direction?
Overall, our analysis allows us to highlight several insights, research gaps, as well as challenges to certain assumptions.
Long-Term Security. Encryption algorithms available today are designed to provide confidentiality for at most 20–30 years. That is, even if no vulnerability is discovered, a highly motivated adversary can start brute forcing all possible decryption keys and will eventually succeed after a couple of decades. This “computational model” is perfectly reasonable in most cases as the sensitivity of (encrypted) data rarely lasts that long: financial records are no longer relevant, classified documents get de-classified, etc. But not really in the context of genomic data. Unfortunately, however, except for one article, all genome privacy proposed solutions do rely on this computational model, ultimately failing to provide any reasonable guarantees of long-term security.
Security Assumptions. Our analysis also indicates that the overwhelming majority of solutions only consider “semi-honest adversaries.” In Cryptography, we differentiate between arbitrary malicious adversaries, for which we make no assumptions as to what they might or might not do, and semi-honest ones, who are assumed to follow protocol and can only passively try to violate privacy. Unsurprisingly, providing security in the fully malicious model is much harder and often yields solutions that are usually much less efficient and flexible, and that do not scale to the extent of fully sequenced genomes and large cohorts. In practice, however, semi-honest assumptions impose important limitations on the real-world security, as, for instance, we cannot make any guarantees as to whether computational tests or patient information have not been maliciously altered or inflated by the adversary.
Non-Collusion. We also observe that a number of solutions involve “third-parties”, and assume that these parties do not collude with other entities. While in certain cases the incentives not to collude are well-reasoned, in other settings, this additional assumption casts another shadow on real-world security implications.
The Cost of Privacy. Unsurprisingly, we find a clear trade-off between privacy protection and utility and flexibility. That is, encryption and other PETs hinder resilience to sequencing errors or, worse yet, limit the capabilities of clinicians and researchers. For example, performing computational genetic testing only over encrypted data (i.e., without seeing the raw data) provides strong privacy guarantees to individuals, but, at the same time, deprives the clinician of the freedom to look at whichever data they might deem useful for diagnostic purposes, or slow down their work. In a trial conducted in Switzerland in 2016, researchers piloted the use of homomorphic encryption to protect genetic markers of HIV-positive patients, allowing their doctors to obtain test results in a privacy-preserving way; alas, the study showed very low acceptability from the physicians.
The loss in utility is even more evident for techniques relying on Differential Privacy (DP) to support statistical research in a privacy-respecting way. In a nutshell, we could use DP to add noise to genomic datasets and hide sensitive attributes, supporting statistical research (e.g., computing the statistical significance of certain genes related to a disease) while minimizing the risk of inferences (e.g., the presence of data of a specific individual in those datasets). Unfortunately, however, solutions presented thus far are limited by this privacy-utility trade-off. That is, in order to get reasonable levels of privacy, one would need to add so much noise that the accuracy of the statistical tasks would ultimately be often destroyed. As the biomedical community strives to achieve as accurate results as possible, it is quite hard to convince them they need to accept a non-negligible utility loss.
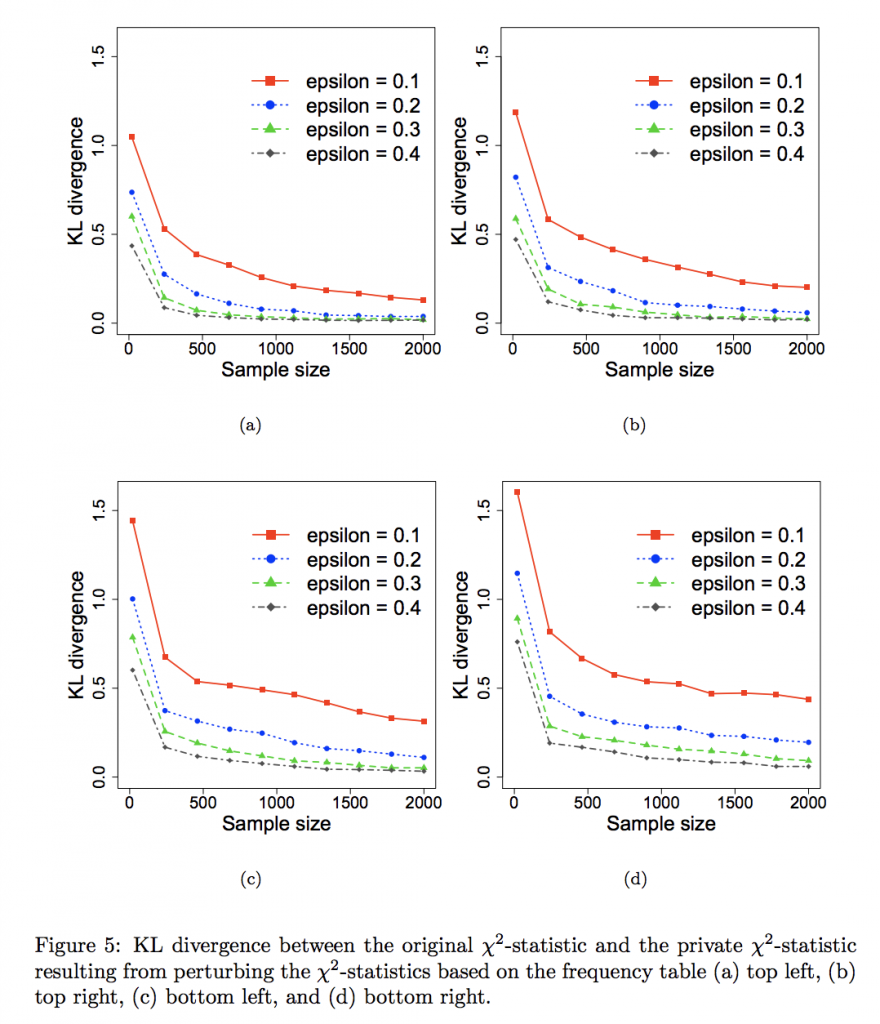
Real-Life Deployability. Finally, we reason about the relevance of genome privacy solutions from a market standpoint. Companies like 23andMe and AncestryDNA offer ancestry and genealogy services directly to consumers, and as their customer base grows, their services become more accurate – e.g., they can match a greater number of relatives. Such companies operate by monetizing their customer’s data, e.g., providing pharmaceutical companies with access to data at a certain cost, which means that without access to their data, their business model is not viable. Therefore, solutions for privacy-preserving ancestry testing that operate over encrypted data are hardly sustainable. On the other hand, however, there might more of a case for health-related genetic tests to be offered (and financially supported) by healthcare providers.
Moreover, proposed solutions are not really applicable to large-scale research initiatives, such as Genomics England or All of Us. Currently, these initiatives deal with privacy using access control mechanisms and informed consent, but ultimately require participants to voluntarily agree to make their genomic information available to any researchers who wish to study it.
Looking Ahead
Although genome privacy is facing a series of non-trivial challenges and limitations, there are cases where privacy-preserving solutions can make a big difference, by enabling genomic research instead of hindering it. For instance, genetic and health data cannot easily cross borders due to legal and policy restrictions. This renders international collaborations very challenging. PETs solutions that allow processing of genomic data in a privacy-preserving way can alleviate such restrictions and enable important genomic research progress.
Overall, we believe that genome privacy research has now reached an important stage. There is a critical mass of leading research groups and technological artifacts that build on PETs to protect genome privacy, although with a number of challenges and obstacles. It is now time to move on to address these challenges and zero in on bringing them out of their cryptography closet and into the real-world.