In the run-up to this year’s Privacy Enhancing Technologies Symposium (PETS 2019), I noticed some decidedly non-privacy-enhancing behaviour. Transport for London (TfL) announced they will be tracking the wifi MAC addresses of devices being carried on London Underground stations. Before storing a MAC address it will be hashed with a key, but since this key will remain unchanged for an extended period (2 years), it will be possible to track the movements of an individual over this period through this pseudonymous ID. These traces are likely enough to link records back to the individual with some knowledge of that person’s distinctive travel plans. Also, for as long as the key is retained it would be trivial for TfL (or someone who stole the key) to convert the someone’s MAC address into its pseudonymised form and indisputably learn that that person’s movements.
TfL argues that under the General Data Protection Regulations (GDPR), they don’t need the consent of individuals they monitor because they are acting in the public interest. Indeed, others have pointed out the value to society of knowing how people typically move through underground stations. But the GDPR also requires that organisations minimise the amount of personal data they collect. Could the same goal be achieved if TfL irreversibly anonymised wifi MAC addresses rather than just pseudonymising them? For example, they could truncate the hashed MAC address so that many devices all have the same truncated anonymous ID. How would this affect the calculation of statistics of movement patterns within underground stations? I posed these questions in a presentation at the PETS 2019 rump session, and in this article, I’ll explain why a set of algorithms designed to violate people’s privacy can be applied to collect wifi mobility information while protecting passenger privacy.
It’s important to emphasise that TfL’s goal is not to track past Underground customers but to predict the behaviour of future passengers. Inferring past behaviours from the traces of wifi records may be one means to this end, but it is not the end in itself, and TfL creates legal risk for itself by holding this data. The inferences from this approach aren’t even going to be correct: wifi users are unlikely to be typical passengers and behaviour will change over time. TfL’s hope is the inferred profiles will be useful enough to inform business decisions. Privacy-preserving measurement techniques should be judged by the business value of the passenger models they create, not against how accurate they are at following individual passengers around underground stations in the past. As the saying goes, “all models are wrong, but some are useful”.
Simulating privacy-preserving mobility measurement
To explore this space, I built a simple simulation of Euston Station inspired by one of the TfL case studies. In my simulation, there are two platforms (A and B) and six types of passengers. Some travel from platform A to B; some from B to A; others enter and leave the station at one platform (A or B). Of the passengers that travel between platforms, they can take either the fast route (taking 2 minutes on average) or the slow route (taking 4 minutes on average). Passengers enter the station at a Poisson arrival rate averaging one per second. The probabilities that each new passenger is of a particular type are shown in the figure below. The goal of the simulation is to infer the number of passengers of each type from observations of wifi measurements taken at platforms A and B.
Assuming the full 48-bit MAC address is recorded, we can see the range of predictions of the size of each passenger population, taken over 100 simulations, (blue) are quite close to the simulation parameters (red).
If, for privacy-protection reasons, we truncate MAC addresses at 16 bits the inferences consistently miss the simulation parameters. Specifically, we underestimate the number of passengers entering and leaving at the same platform and overestimate those moving between platforms. This inaccuracy is a consequence of the simple-minded matching algorithm that just looks at the first occurrence of a MAC address at a location. Two devices with MAC addresses that happen to truncate to the same value, that each stay at one location, will be inferred to be one device that moves between locations. This “ghost trace” is inferred even if the movement doesn’t make sense because it is implausibly fast or even that it goes backwards in time.
Can we do better? Certainly – we can take into account background knowledge about what types of movements are plausible when matching individual MAC addresses. We can also look at the matching as a whole to ensure each MAC address that enters a station is linked to exactly one that leaves (a perfect match, in graph theory). Efficiently performing this match is hard, but fortunately, there is a whole field of information security which has developed techniques for doing so – mix network de-anonymisation.
Applying mix-network de-anonymisation for good
A mix network takes in several messages, encrypts them, and sends them out again in a random order after a delay. The goal of the network is to hide the correspondence between input messages and output messages to protect the privacy of senders and receivers. However, it has long been known that mix networks leak information as a result of how the mix is designed, e.g. mixes cannot send messages before they are received and some delay periods are more likely than others. Mixes also leak information due to how people use them, e.g. senders likely have a few favourite communication partners and a message sent is possibly going to elicit a reply.
Researchers working in the field of mix-network de-anonymisation have developed algorithms that allow an adversary to take this information leakage and other background knowledge and identify the most likely senders and recipients for each message1. As an intermediate step, these algorithms infer profiles of users, indicating preferred communication partners and other behaviours. Often this profile is of more interest than the messages themselves because it can predict future behaviour and so allows an adversary to identify which users of the mix are of most interest and so should be subject to more detailed investigation.
The central observation of my talk is that these mix-network de-anonymisation algorithms, designed to allow an adversary to violate the privacy of network users, can be applied for good – to facilitate privacy-preserving prediction of mobility patterns. A mix-network protects outgoing messages by arranging that each could potentially be the result of many different incoming messages. Privacy-preserving wifi measurement protects individuals by truncating the MAC address such that many potential devices may have caused a particular event. Mix-network de-anonymisation algorithms infer profiles of user’s messaging behaviour. In the context of privacy-preserving wifi measurement, the same algorithm can infer profiles of users’ mobility behaviour.
A Bayesian approach to mobility profile inference
For example, consider Vida, a very general mix-network de-anonymisation algorithm. Observations (O) of a mix network are the result of the sender and recipient of each message (M), how these messages are assigned to routes through the anonymity system (Φ), and the constraints of the anonymity system design (A). Sender-recipient pairs (M) in turn, depend on user profiles (Ψ). Vida takes a Bayesian approach to infer user profiles (Ψ) given knowledge of the anonymity system design (A), routing behaviour (Φ), and a set of observations (O).
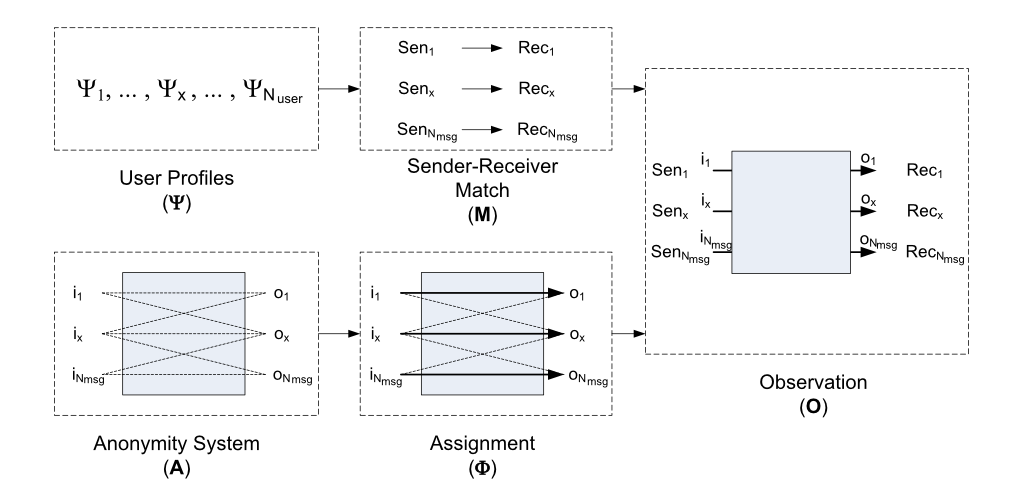
In the context of privacy-preserving wifi measurement, observations (O) are a result of how users move through the station (M), the anonymisation scheme (Φ), and the physical constraints of the stations (A). User movements, in turn, depend on user mobility profiles (Ψ). A variation of Vida applied to infer user mobility profiles (Ψ) could do so given the observations (O), a definition of the anonymisation scheme (Φ), and knowledge of the station and wifi measuring network (A).
By simulating wifi measurements taken from devices moving around stations, we could explore the safest level of anonymisation that would result in mobility profiles of sufficient confidence to base business decisions. These simulations should also take into account other limitations of the measurement approach affecting the accuracy, such as the bias introduced by only measuring customers with wifi-enabled devices and variation of customer behaviour over time. If a particular business decision is very sensitive to the level of anonymisation, it is quite likely to be sensitive to other limitations of the dataset, and so cannot be answered confidently regardless of anonymisation approach.
The purpose of this article is to present the idea of applying mix-network de-anonymisation algorithms to privacy-preserving measurement. I would also claim this article shows that it is possible to measure mobility patterns without violating the privacy of travellers. There are many directions this work could be taken. For example, I selected MAC address truncation because it is simple to explain and could be trivially implemented, but this approach only causes several users to be seen as one – never one user as several. An alternative would be to set some bits at random, which would allow both possibilities. It might even be possible to apply a similar approach to infer information about user behaviour when there’s address randomisation built into end-devices, such as with mobile devices and IPv6.
Feel free to play around with the simulation and if you have any of your own ideas, do let me know in the comments.
1 Although I’ve phrased de-anonymisation algorithms as being applied by adversaries and jokingly called the presentation “de-anonymisation techniques for good”, the vast majority of academic researchers in this field are working to improve the security of mix networks. To do so, they develop better de-anonymisation algorithms and share these with others at events like PETS so that the level of security that mix networks provide is better understood and to help practitioners develop future generations of mix networks are resistant to such attacks.